Creating unbiased, accurate algorithms isn’t impossible — it’s just time consuming.
“It actually is mathematically possible,” facial recognition startup Kairos CEO Brian Brackeen told me on a panel at TechCrunch Disrupt SF.
Algorithms are sets of rules that computers follow in order to solve problems and make decisions about a particular course of action. Whether it’s the type of information we receive, the information people see about us, the jobs we get hired to do, the credit cards we get approved for, and, down the road, the driverless cars that either see us or don’t, algorithms are increasingly becoming a big part of our lives. But there is an inherent problem with algorithms that begins at the most base level and persists throughout its adaption: human bias that is baked into these machine-based decision-makers.
Creating unbiased algorithms is a matter of having enough accurate data. It’s not about just having enough “pale males” in the model, but about having enough images of people from various racial backgrounds, genders, abilities, heights, weights and so forth.
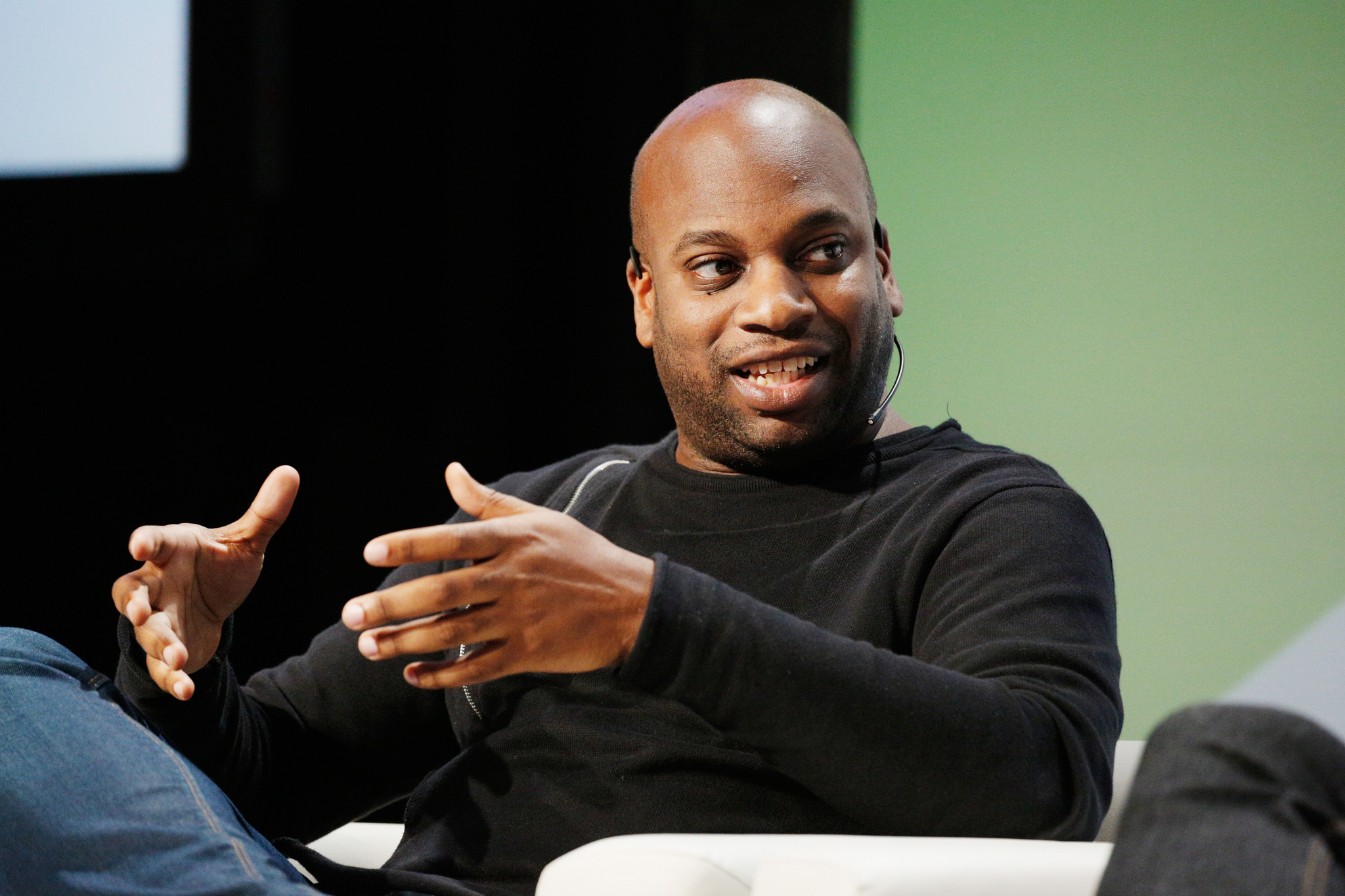
Kairos CEO Brian Brackeen
“In our world, facial recognition is all about human biases, right?” Brackeen said. “And so you think about AI, it’s learning, it’s like a child and you teach it things and then it learns more and more. What we call right down the middle, right down the fair way is ‘pale males.’ It’s very, very good. Very, very good at identifying somebody who meets that classification.”
But the further you get from pale males — adding women, people from different ethnicities, and so forth — “the harder it is for AI systems to get it right, or at least the confidence to get it right,” Brackeen said.
Still, there are cons to even a one hundred percent accurate model. On the pro side, a good facial recognition use case for a completely accurate algorithm would be in a convention center, where you use the system to quickly identity and verify people are who they say they are. That’s one type of use case Kairos, which works with corporate businesses around authentication, addresses.
“So if we’re wrong, at worst case, maybe you have to do a transfer again to your bank account,” he said. “If we’re wrong, maybe you don’t see a picture accrued during a cruise liner. But when the government is wrong about facial recognition, and someone’s life or liberty is at stake, they can be putting you in a lineup that you shouldn’t be in. They could be saying that this person is a criminal when they’re not.”
But in the case of law enforcement, no matter how accurate and unbiased these algorithms are, facial recognition software has no business in law enforcement, Brackeen said. That’s because of the potential for unlawful, excessive surveillance of citizens.
Given the government already has our passport photos and identification photos, “they could put a camera on Main Street and know every single person driving by,” Brackeen said.
And that’s a real possibility. In the last month, Brackeen said Kairos turned down a government request from Homeland Security, seeking facial recognition software for people behind moving cars.
“For us, that’s completely unacceptable,” Brackeen said.
Another issue with 100 percent perfect mathematical predictions is that it comes down to what the model is predicting, Human Rights Data Analysis Group lead statistician Kristian Lum said on the panel.
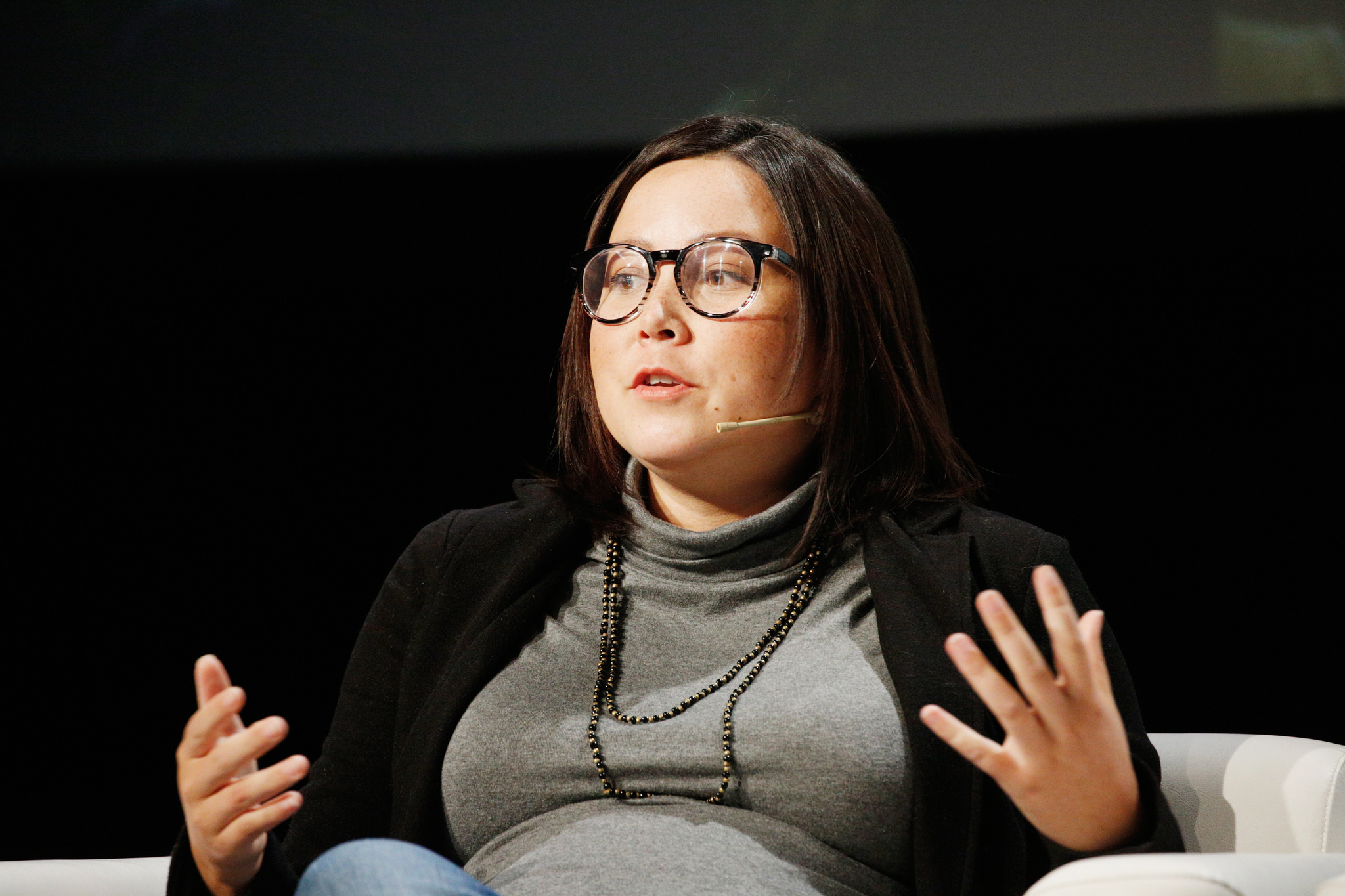
Human Rights Data Analysis Group lead statistician Kristian Lum
“Usually, the thing you’re trying to predict in a lot of these cases is something like rearrest,” Lum said. “So even if we are perfectly able to predict that, we’re still left with the problem that the human or systemic or institutional biases are generating biased arrests. And so, you still have to contextualize even your 100 percent accuracy with is the data really measuring what you think it’s measuring? Is the data itself generated by a fair process?”
HRDAG Director of Research Patrick Ball, in agreement with Lum, argued that it’s perhaps more practical to move it away from bias at the individual level and instead call it bias at the institutional or structural level. If a police department, for example, is convinced it needs to police one neighborhood more than another, it’s not as relevant if that officer is a racist individual, he said.
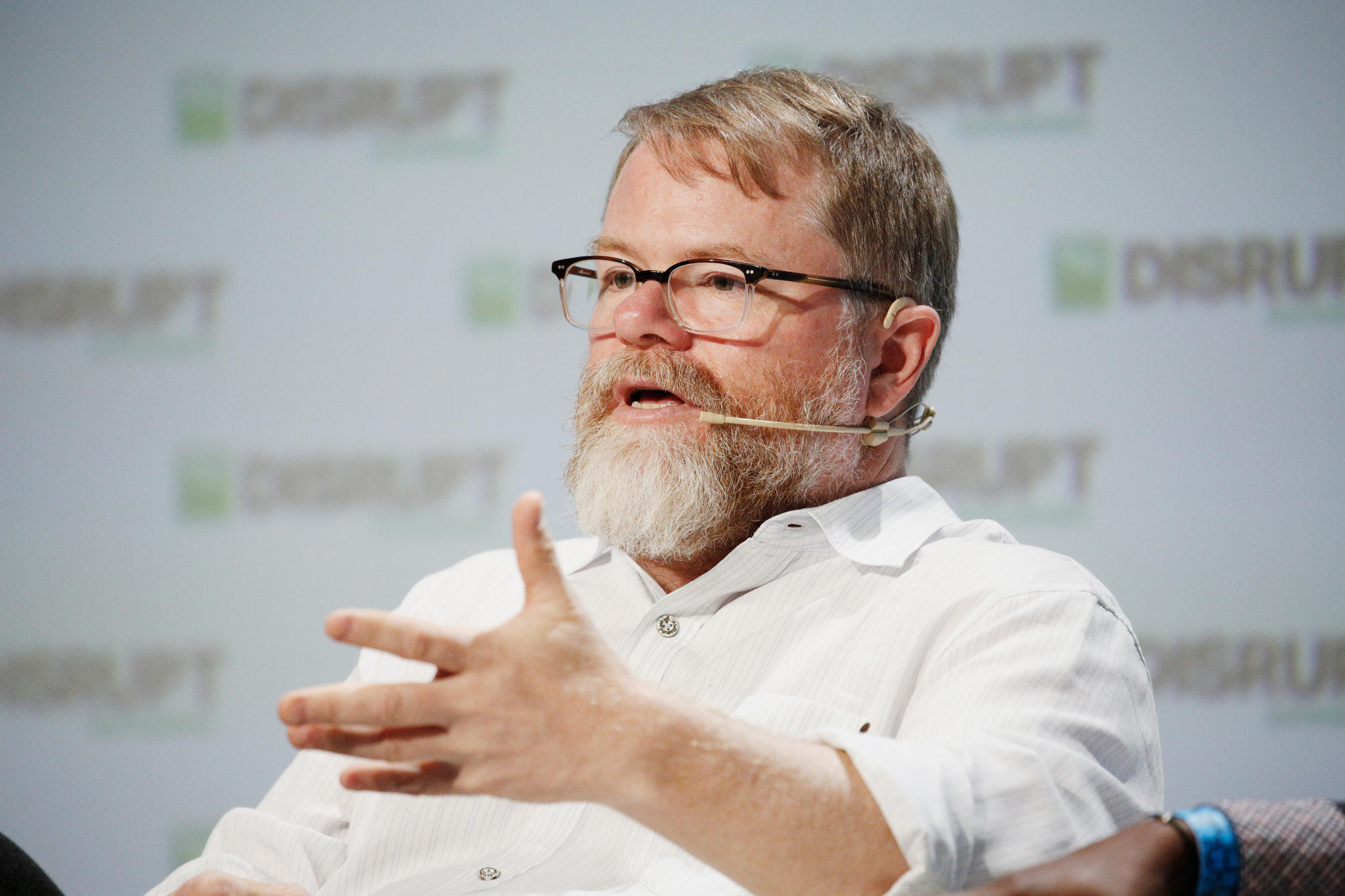
HRDAG Director of Research Patrick Ball
“What’s relevant is that the police department has made an institutional decision to over-police that neighborhood, thereby generating more police interactions in that neighborhood, thereby making people with that ZIP code more likely to be classified as dangerous if they are classified by risk assessment algorithms,” Ball said.
And even if the police were to have perfect information about every crime committed, in order to build a fair machine learning system, “we would need to live in a society of perfect surveillance so that there is absolute police knowledge about every single crime so that nothing is excluded,” he said. “So that there would be no bias. Let me suggest to you that that’s way worse even than a bunch of crimes going free. So maybe we should just work on reforming police practice and forget about all of the machine learning distractions because they’re really making things worse, not better.”
He added, “For fair predictions, you first need a fair criminal justice system. And we have a ways to go.”
from TechCrunch https://ift.tt/2OXXCYP
No comments:
Post a Comment